What Makes Vehicle Market Data Clean - and Why It Matters
Hermes Data | December 25th 2025
What is Automotive Market Data?
In the automotive world, dealers and buyers always want to know the 'right' price. A dealer who is buying a trade in, and the buyer upgrading to a newer year model both do a ton of homework before any purchase.
So how do we know that 'right' price?
All of the big players in the automotive space use what's called automotive market data (or retail data) to compare prices. What that means is looking at all of the cars for sale in the city, state, region, or country to try decide what the 'right' price is.
If 5 dealerships in a city are all consistently selling 2025 Ford Broncos for $50,000, then that's probably the 'right' price. If one dealer starts selling it for $40,000, then they're going to sell it fast - they're below market price. If one dealer tries selling it for $60,000, then they could have to wait a while - they are above market price.
So we can see that if we know all of the recently sold and available cars, we could make good guesses of what dealers are selling for and what buyers are willing to pay. That's the power of automotive market data.
But what if the data is wrong...? Now we have a big problem.
The Truth About Automotive Market Data
The truth about automotive market data is that it is messy. Very messy. It is based on real, organic dealership website data. From your local corner lot, to national dealer group websites.
And because they can post their own listings, there is no standard format for the data. One dealer might misspell a model name, another might not put their full address, and many put 'Call For Price' instead of their actual list price. From the countless retail listings we've reviewed, we've seen it all - even putting directions from the airport as their address.
These seem like minor inconveniences, but they can affect vehicle pricing drastically. Imagine losing 1-10% of your yearly revenue because of missed data...
However, there are some strategies we can use to repair and salvage the data. Let's start with the most important part - price.
Bad Pricing
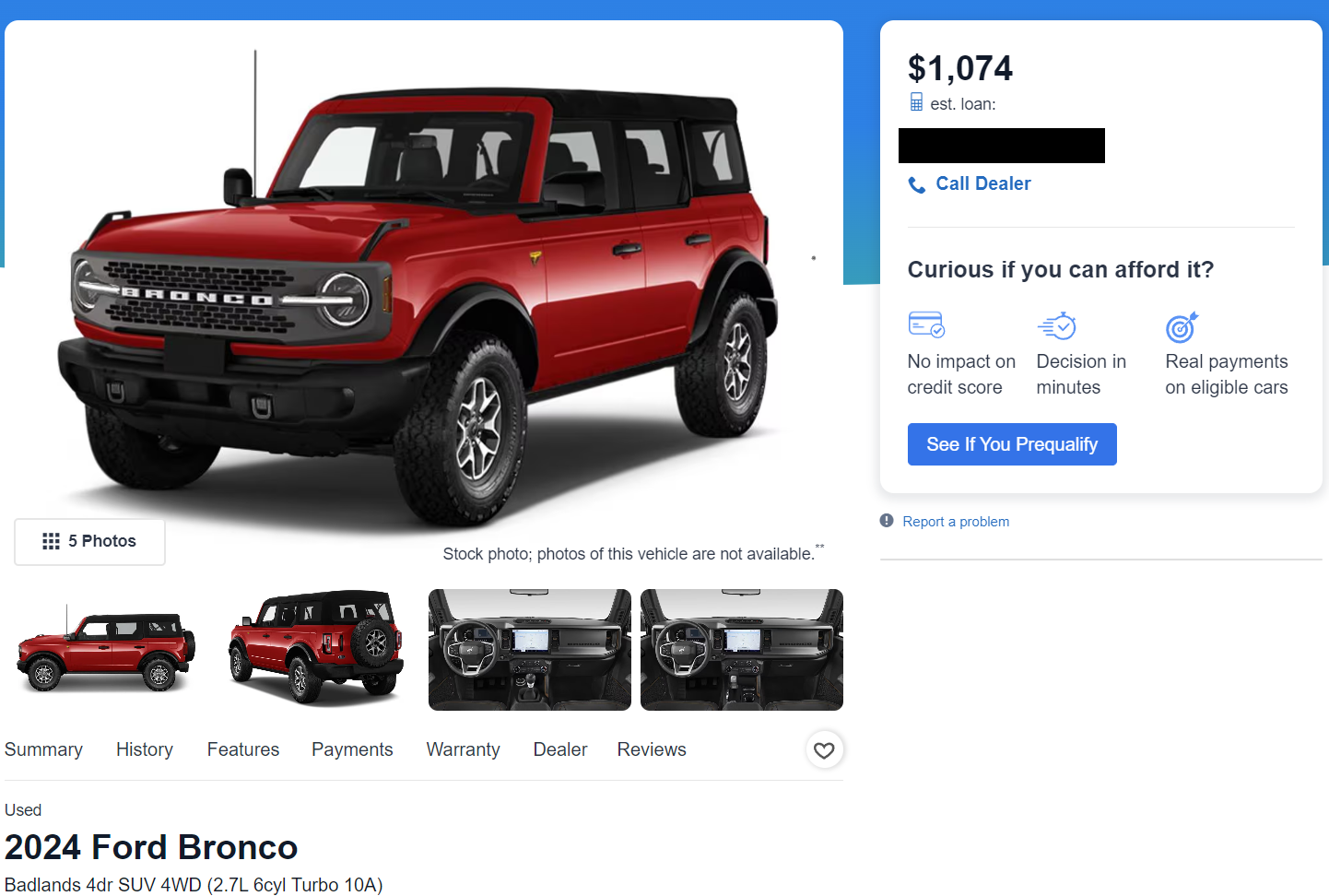
Here we can see a 2024 Ford Bronco priced at $1,074 by a licensed Ford dealer. This price seems too good to be true. It's almost definitely estimated monthly payment, not the actual price.
So what can we do about it? We can fix this with one of any statisical approaches to remove data that is too far from the main group.
Imagine we had hundreds of 2024 Ford Broncos listed around $45,000, then one listed at $1,000 and one listed at $1,000,000. Without getting into the math, you and I know we should cut out the $1,000 and the $1,000,000 data points and just look at those around $45,000.
If you aren't filtering outlier data, your pricing could be what's causing your days-on-lot to be so high.Or on the other side, what's costing you thousands per car of missed revenue.
Incorrect Addresses
Next let's cover the address issue. There is one correct address format, and millions of ways to mess it up.
We've got a few problems here. Primarily, we don't have the city or state. Secondly we have to somehow get 123 Main Street, and the zip code 55555 out from this extra text.
There are many software tools available to try and pull a full address out of scattered text - with varying success. The ultimate solution would be to use a geocoding service with the dealer name, like you or I would do with a Google search.
We require location data with every listing because every location has its own unique market. We all know that luxury car is more valuable in Beverly Hills than on the nothern coast of Canada. We need to know where the car is to know how much it's worth.
*The actual address has been edited for the dealer's privacy, but it is based on reality.
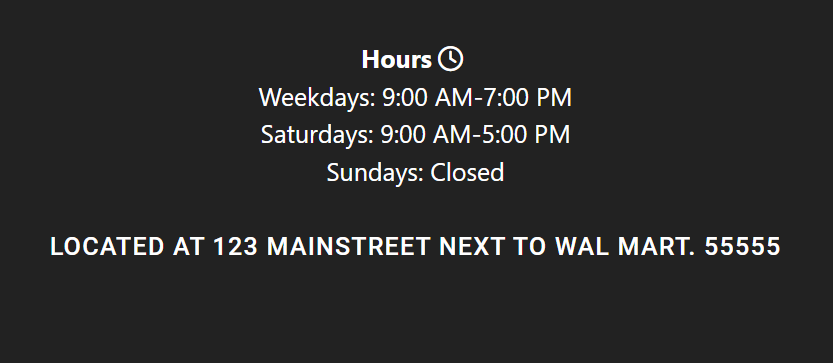
Normalized Make/Model/Trim

I didn't know Corvette had such a model...
This is one of the hardest problems to solve in cleaning retail data. There is so much extra data in the vehicle heading - even with advanced AIs you're going to have a hard time getting the right model and trim.
One approach is to source all of the current makes/models/trims from the manufacturer and compare with that list. If the heading has 'Chevrolet', 'Corvette', 'Z06', and '1LZ', we can reasonably say we've found a match.
This method works most of the time. But it assumes the dealer will spell everything correctly. After all, what obligation do they have to make our data collection easier? Their goal is to sell cars to customers.
If you don't have the correct year, make, model and trim - how could you possibly compare how each manufacturer is performing? Or how different models and trims are competing in the same segment?
Conclusion
So let's ask the question again - why does clean data matter?
One missed price here, a missed address there, or a missed upgrade package can cost you thousands of dollars per car. And if you're selling hundreds of cars per month, you're looking at millions per year of missed revenue.
For the dealers out there, make sure your partner services are working off of clean, complete data.
For the dealership serving companies out there, consider the disservice you could be doing to your clients with subpar data.
Thanks for reading our article.
If you found value in this, consider sharing it with your data departments and make sure your data is clean and correct.
You could be costing yourselves and your clients millions per year.